Special Session at IEEE International Symposium on Biomedical Imaging 2022
March 31st 2022
8:30 AM - 10:30 AM
Biomedical Imaging for Equitable Deep Learning, Regulatory Science, and Clinical Research
Session Summary
Tools, models and statistical methods for signal processing and medical image analysis and training deep learning models to create research prototypes for eventual clinical applications are of special interest to the biomedical community. But material and optical properties of biological tissues are complex and not easily captured by imaging devices. Added complexity can be introduced by biased datasets with underrepresentation of medical images from races and ethnicities for deep learning, and limited knowledge about regulatory framework needed for commercialization and safety of emerging technologies using image analysis. This special session describes major topics and opportunities for validating capture devices and medical images of skin (RGB), prostate tissues (digital pathology) and lungs and kidneys (grayscale) for clinical diagnosis. Strategies and methods for training of unbiased, high performance locked and adaptive deep learning models with uncertainty quantifications will be discussed. Special emphasis is made on the integration of unbiased imaging data and its positive impact by engendering fairness in deep learning model performance. Additionally, speakers from the United States Food and Drug Administration (FDA) share guidance for an evolving regulatory framework for software-based medical devices powered by deep learning.
Conference Paper
Jenna Lester, Delfino J, Pai V, Shah P*. "Biomedical imaging for equitable deep learning, regulatory science and clinical research." IEEE International Symposium on Biomedical Imaging, 31 Mar. 2022, special session. [Paper]
(*Senior author conceptualizing and leading the session)
Talks
Topic: Biomedical Imaging & Capture for Uncertainty-quantified Deep Learning
Speaker: Pratik Shah*. Ph.D.
Dr. Pratik Shah is the Principal Investigator leading the Health 0.0 research lab at MIT. He conceptualizes and leads this special session by opening with recent advances in uncertainty quantified deep learning architectures and statistical tools from his lab that can be used for investigating the real-world performance of deep learning models for bias, equity and fairness.
Topic: Medical Imaging for Diversity, Equity, and Inclusion for Skin Tones
Speaker: Jenna Lester. MD
Jenna Lester, MD is a dermatologist and assistant professor in the Department of Dermatology at the School of Medicine in the University of California, San Francisco. Dr. Lester speaks about expanding the clinical features of medical images to include balanced datasets with different races, ethnicities, and skin tones as rapidly emerging field to train unbiased and fair deep learning systems.
Topic: Regulatory Perspectives on AI/ML enabled Medical Devices
Speaker: Jana Delfino. Ph.D.
Jana G. Delfino, PhD, is the Deputy Director for Medical Imaging and Digital Health in FDA’s Division of Diagnostics, Imaging, and Software Reliability in the Office of Science and Engineering Laboratories. Dr. Delfino provides insights into FDA’s regulatory review process for the medical image processing, deep learning and signal processing communities working on real-world clinical applications of their technologies.
Topic: From Models to Real-world Performance
Speaker: Vinay M. Pai. Ph.D., MBA
Vinay Pai is a General Engineer in FDA’s Center for Devices and Radiological Health (CDRH), Office of Strategic Partnerships and Technology Innovation (OST), Division of Digital Health (DDH). Vinay will share solutions for challenged faced by medical devices that incorporate AI/ML technologies and imaging devices, models, and products once they reach the patient or healthcare provider will be discussed.
Special session leader: Pratik Shah. Ph.D.
Pratik Shah Ph.D. is a lab head and principal investigator at MIT. He leads a research lab at MIT that works on novel scientific discoveries powered by medical imaging, machine learning, and biological technologies to improve clinical, regulatory science and health outcomes in patients. His work builds an unified scaffolding of novel deep learning, biological and statistical reasoning methods to resolve distinct hypothesis driven research problems including, for example, how to diagnose and treat cancer disease, how to leverage causal structures in big observational data for unbiased patient centered medicine, and how infectious microbes and host cells adapt during disease – under a single theoretical and methodological framework. Recent work from his lab has been published in Nature Digital Medicine, Cell Reports Methods, JAMA, IEEE conferences, and Proceedings of National Academies of Science Engineering and Medicine workshops. Pratik has BS, MS, and PhD degrees in biological sciences and completed fellowship training at Massachusetts General Hospital, the Broad Institute of MIT and Harvard, and Harvard Medical School.
Contact Information
Massachusetts Institute of Technology
20 Ames Street
E15, 392
Cambridge, MA 02139
pratiks@mit.edu
Speakers
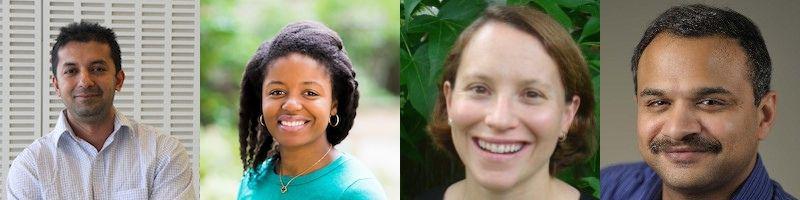
References
[1] S. Ghosal, and P. Shah “A deep-learning toolkit for visualization and interpretation of segmented medical images,” Cell Reports Methods, vol. 1, no. 7, pp.100107, November, 2021.
[2] Rana, A., Lowe, A., Lithgow, M., Horback, K., Janovitz, T., Da Silva, A., Tsai, H., Shanmugam, V., Bayat, A., & Shah, P. (2020). Use of Deep Learning to Develop and Analyze Computational Hematoxylin and Eosin Staining of Prostate Core Biopsy Images for Tumor Diagnosis. JAMA network open, 3(5), e205111. https://doi.org/10.1001/jamanetworkopen.2020.5111
[3] Shah, P., Kendall, F., Khozin, S., Goosen, R., Hu, J., Laramie, J., Ringel, M., & Schork, N. (2019). Artificial intelligence and machine learning in clinical development: a translational perspective. NPJ digital medicine, 2, 69. https://doi.org/10.1038/s41746-019-0148-3
[4] Lester, J. C., Jia, J. L., Zhang, L., Okoye, G. A., & Linos, E. (2020). Absence of images of skin of color in publications of COVID-19 skin manifestations. The British journal of dermatology, 183(3), 593–595. https://doi.org/10.1111/bjd.19258
[5] Lester, J. C., Taylor SC. Resisting racism in dermatology: A call to action. JAMA Dermatol. 2021 Mar 1;157(3):267-268. doi: 10.1001/jamadermatol.2020.5029.
[6] Submission of premarket notifications for magnetic resonance diagnostic devices: Guidance for industry and Food and Drug Administration Staff. Final issued November 18, 2016; draft July 14, 2015. (Delfino, J)
[7] Pearlman, P. C., Divi, R., Gwede, M., Tandon, P., Sorg, B. S., Ossandon, M. R., Agrawal, L., Pai, V., Baker, H., & Lash, T. B. (2016). The National Institutes of Health Affordable Cancer Technologies Program: Improving Access to Resource-Appropriate Technologies for Cancer Detection, Diagnosis, Monitoring, and Treatment in Low- and Middle-Income Countries. IEEE journal of translational engineering in health and medicine, 4, 2800708.
[8] U.S. Food and Drug Administration. Digital Health Software Precertification (Pre-Cert) Program. https://www.fda.gov/medical-devices/digital-health- center-excellence/digital-health-software- precertification-pre-cert-program
[9] U.S. Food and Drug Administration. Digital Health Innovation Action https://www.fda.gov/media/106331/download (2017).